Adaptive Training (AT) is transforming how we approach learning and development. By utilising data and technology, AT personalises educational experiences to align with individual learners’ needs, abilities, and preferences. This innovative method is designed to accelerate learning and enhance its effectiveness, enabling trainees to develop expertise more rapidly. But how does adaptive training work? What is the evidence behind it, and is it truly effective?
In this article, we’ll explore the fundamentals of AT, chart its evolution, and highlight how cutting-edge technologies, such as eye tracking and extended reality, are shaping its future.
What is Adaptive Training?
First introduced by Charles Kelley in 1969, AT refers to a learning approach where tasks or problems adjust based on a trainee’s performance. Put simply, tasks begin at a level that matches the learner’s current ability and gradually becomes more complex as they improve.
Initially, AT relied on human instructors who assessed learners’ verbal and non-verbal cues and tailored their teaching accordingly. For example, if a student displayed confusion or struggled with performance, the instructor could simplify tasks or modify their teaching style. Research by Bloom (1984) found that this personalised, one-to-one tutoring model resulted in a two standard deviation improvement in student performance compared to traditional classroom settings—a phenomenon known as the “2 sigma problem.”
The Challenge of Scalability
While highly effective, Bloom’s research found one-to-one AT to be time-consuming and costly, making it impractical for large-scale applications. This limitation prompted researchers to explore how technology could replicate the adaptability of human instructors. Enter computer-supported training systems, which monitor learner performance and dynamically adjust training tasks in real-time. These systems made it scalable, cost-effective, and accessible to larger groups.
The Evolution of Adaptive Training
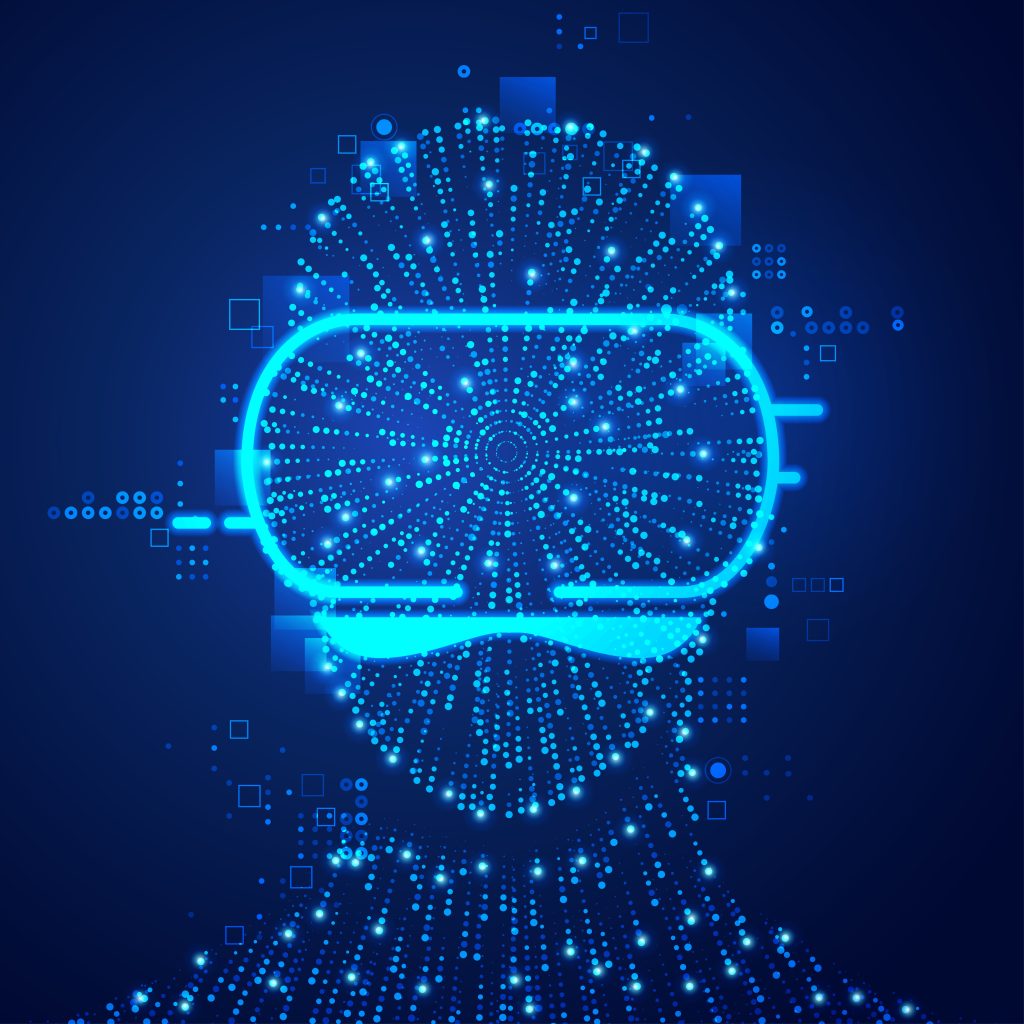
Over the past three decades, researchers have developed several distinct approaches to AT, including:
- Macro-Adaptation: Training is tailored before the session begins, adjusting instructional goals, delivery or depth of content based on the learner’s general abilities or achievement level.
- Aptitude Treatment Interaction (ATI): Content is matched to a learner’s aptitudes and abilities before training.
- Micro-Adaptation: Training dynamically adjusts in real-time based on the learner’s ongoing performance.
- Hybrid Approaches: A combination of ATI and micro-adaptation, where training initially adapts to aptitudes and later transitions to real-time performance adjustments as more data becomes available.
Across these methods, AT employs algorithms to modify task difficulty dynamically, a process commonly referred to as dynamic difficulty adjustment.
Adaptive Training in Action
Modern adaptive training systems integrate advanced tools such as virtual reality, eye tracking, and even brain activity monitoring to assess and respond to trainee performance. Below are a few notable studies demonstrating their effectiveness:
- Van Buskirk et al. (2014): Explored the effectiveness of micro-adaptive and hybrid training approaches.
- Dey et al. (2019): Demonstrated an EEG-based adaptive training system in virtual reality.
- Aguilar Reyes et al. (2023): Evaluated the design and impact of an adaptive virtual reality training system.
- Finseth et al. (2024): Highlighted how adaptive stress exposure training outperformed non-adaptive methods for stress inoculation.
Cineon’s Adaptive Training Approach
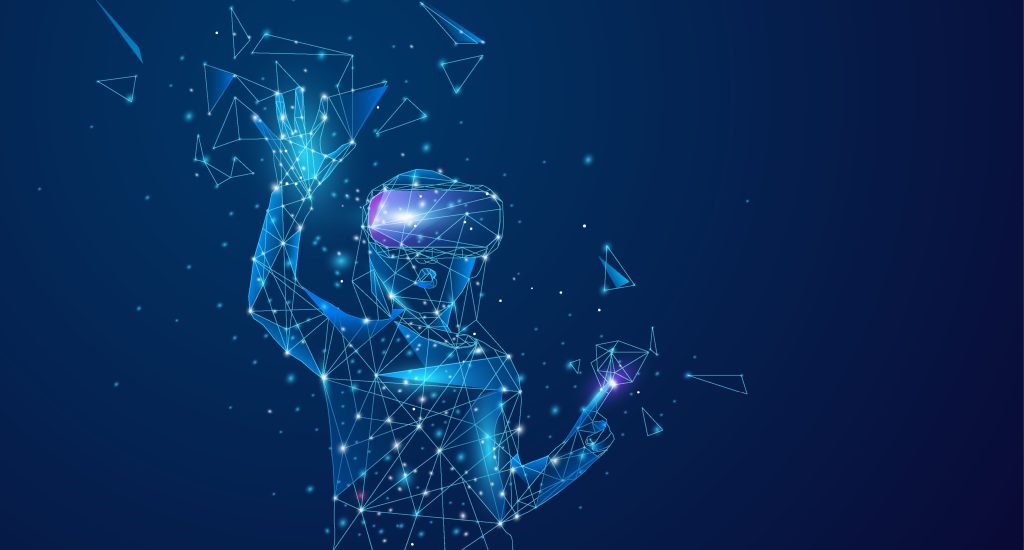
At Cineon, we have taken adaptive training to the next level by leveraging Extended Reality (XR) and eye-tracking technology. Here’s how we ensure our approach is both effective and seamless:
1. Eye-Tracking Technology
The biggest challenge in adaptive training is accurately monitoring and interpreting trainee performance in real-time. Eye tracking provides a lightweight, unobtrusive solution for collecting data on cognitive load, attention, and emotional states.
2. Data Analysis Pipeline
The raw data captured by eye trackers has to be processed and interpreted to enable meaningful adaptations. At Cineon, we’ve developed a robust data pipeline that transforms sensor inputs into actionable insights, allowing training programmes to adapt dynamically to the learner’s needs.
3. Scalable Solutions
Our API, Empathic Learning Engine (ELE), integrates seamlessly with existing training systems, enabling adaptive features to be implemented across industries—from aviation to healthcare and beyond.
The Future of Adaptive Training
Adaptive training is more than just a buzzword—it’s a transformative approach that uses technology to unlock human potential. With advancements in sensors, algorithms, and immersive technologies, adaptive training will continue to evolve, providing scalable solutions across education, defence, healthcare, and other industries.
As Cineon leads the charge in adaptive training innovation, we’re committed to helping individuals and organisations achieve their goals faster, more efficiently, and with unmatched precision.
Ready to unlock human potential?
Contact our team to discuss how Cineon’s XR solutions, eye-tracking and API can help you get the full human performance picture.
References
Bloom, B. S. (1984). The 2 Sigma Problem: The Search for Methods of Group Instruction as Effective as One-to-One Tutoring. Educational Researcher, 13(6), 4-16.
Finseth, T., Dorneich, M. C., Keren, N., Franke, W. D., & Vardeman, S. (2024). Virtual Reality Adaptive Training for Personalized Stress Inoculation. Human Factors, 0(0). https://doi.org/10.1177/00187208241241968.
Van Buskirk, W. L., Steinhauser, N. B., Mercado, A. D., Landsberg, C. R., & Astwood, R. S. (2014). A Comparison of the Micro-Adaptive and Hybrid Approaches to Adaptive Training. Proceedings of the Human Factors and Ergonomics Society Annual Meeting, 58(1), 1159-1163. https://doi.org/10.1177/1541931214581242.
A. Dey, A. Chatburn and M. Billinghurst, “Exploration of an EEG-Based Cognitively Adaptive Training System in Virtual Reality,” 2019 IEEE Conference on Virtual Reality and 3D User Interfaces (VR), Osaka, Japan, 2019, pp. 220-226, doi: 10.1109/VR.2019.8797840.
Aguilar Reyes, C.I., Wozniak, D., Ham, A. et al. Design and evaluation of an adaptive virtual reality training system. Virtual Reality 27, 2509–2528 (2023). https://doi.org/10.1007/s10055-023-00827-7